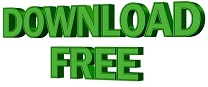
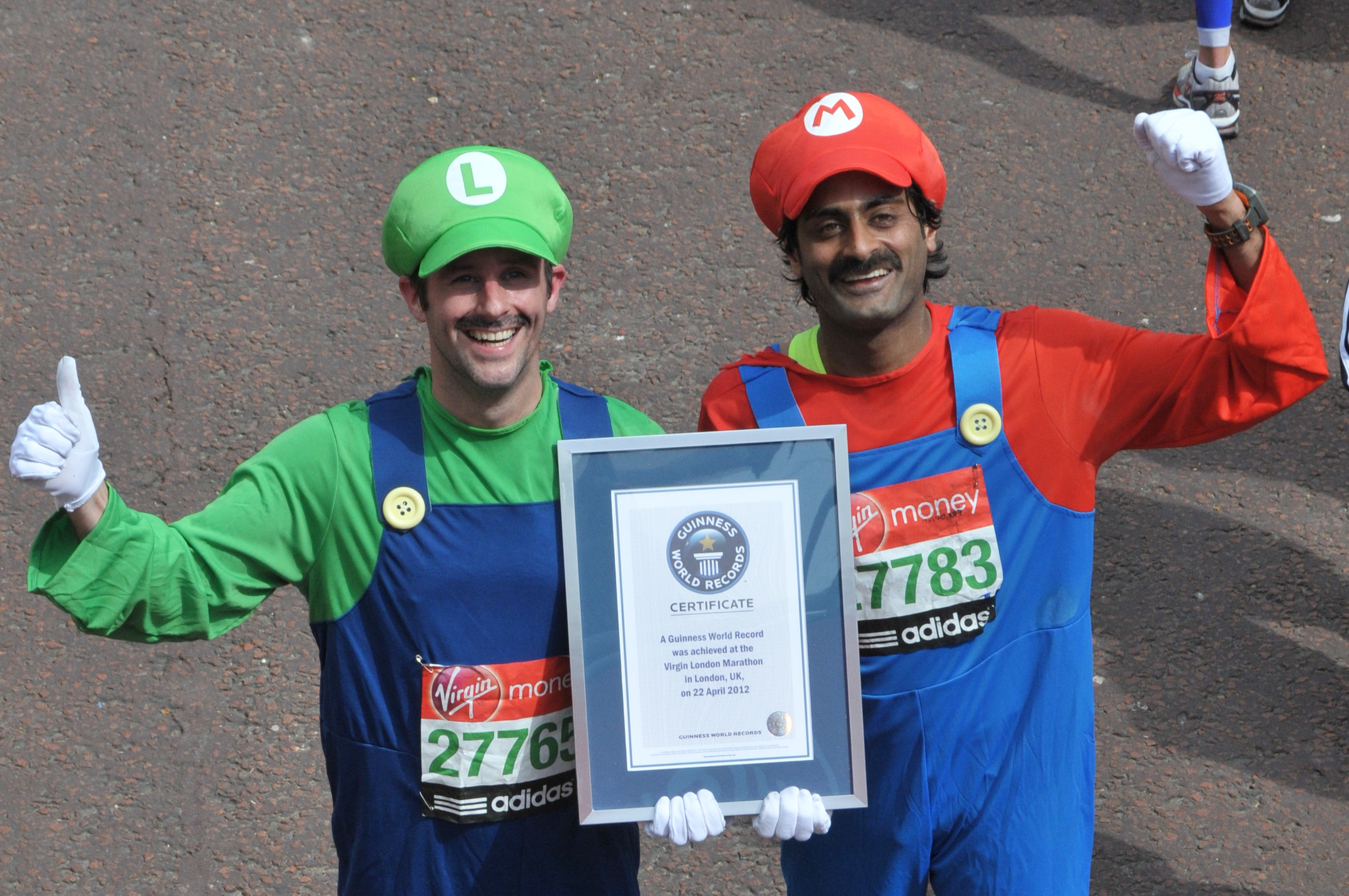
Unlike other proposed approaches, our real-world experiments demonstrate successful task learning from raw visual input on a fully actuated robot manipulator. We present an early demonstration of this approach with a number of experiments in the domain of robot manipulation that focus on bridging the reality gap. The progressive net approach is a general framework that enables reuse of everything from low-level visual features to high-level policies for transfer to new tasks, enabling a compositional, yet simple, approach to building complex skills. We propose using progressive networks to bridge the reality gap and transfer learned policies from simulation to the real world. Deep Reinforcement Learning algorithms are too slow to achieve performance on a real robot, but their potential has been demonstrated in simulated environments. We show new state-of-the-art results across multiple adaptation tasks, including digit classification and semantic segmentation of road scenes demonstrating transfer from synthetic to real world domains.Īpplying end-to-end learning to solve complex, interactive, pixel-driven control tasks on a robot is an unsolved problem. Our model can be applied in a variety of visual recognition and prediction settings.
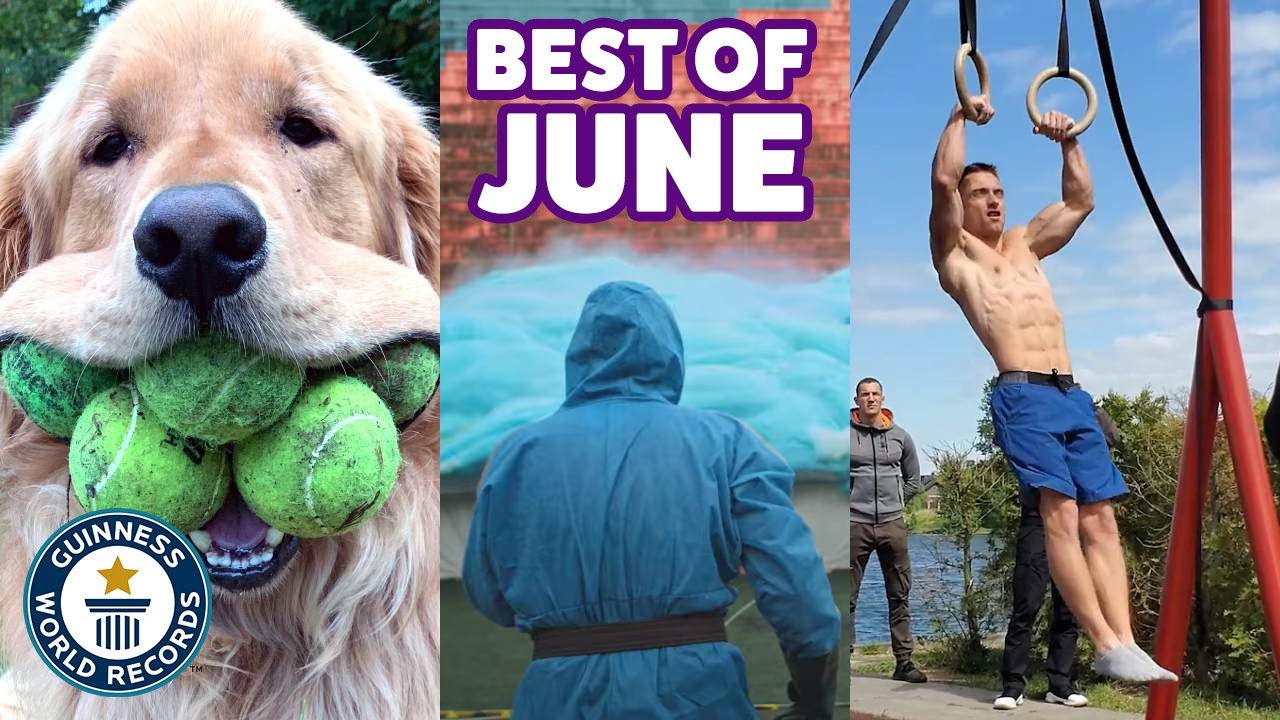
C圜ADA adapts representations at both the pixel-level and feature-level, enforces cycle-consistency while leveraging a task loss, and does not require aligned pairs. We propose a novel discriminatively-trained Cycle-Consistent Adversarial Domain Adaptation model. Recent work has shown that generative adversarial networks combined with cycle-consistency constraints are surprisingly effective at mapping images between domains, even without the use of aligned image pairs. Adversarial adaptation models applied in feature spaces discover domain invariant representations, but are difficult to visualize and sometimes fail to capture pixel-level and low-level domain shifts. Furthermore, it shows promising results on standard domain adaptation benchmarks.ĭomain adaptation is critical for success in new, unseen environments. Our method can successfully transfer navigation policies between drastically different simulators: ViZDoom, SuperTuxKart, and CARLA. We use these recognition datasets to link up a source and target domain to transfer models between them in a task distillation framework. They exist in any interesting domain, simulated or real, and are easy to label and extend.

Our core observation is that for certain tasks, such as image recognition, datasets are plentiful. However, this transfer is challenging since simulated and real-world visual experiences vary dramatically.

The commonly prescribed solution is simple: learn a representation in simulation and transfer it to the real world. Repeatedly crashing a car into a tree is simply too expensive. Unfortunately, tasks like autonomous driving have virtually no real-world training data. Deep networks devour millions of precisely annotated images to build their complex and powerful representations.
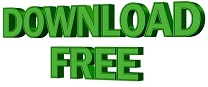